Disciplines
- MLA
- APA
- Master's
- Undergraduate
- High School
- PhD
- Harvard
- Biology
- Art
- Drama
- Movies
- Theatre
- Painting
- Music
- Architecture
- Dance
- Design
- History
- American History
- Asian History
- Literature
- Antique Literature
- American Literature
- Asian Literature
- Classic English Literature
- World Literature
- Creative Writing
- English
- Linguistics
- Law
- Criminal Justice
- Legal Issues
- Ethics
- Philosophy
- Religion
- Theology
- Anthropology
- Archaeology
- Economics
- Tourism
- Political Science
- World Affairs
- Psychology
- Sociology
- African-American Studies
- East European Studies
- Latin-American Studies
- Native-American Studies
- West European Studies
- Family and Consumer Science
- Social Issues
- Women and Gender Studies
- Social Work
- Natural Sciences
- Anatomy
- Zoology
- Ecology
- Chemistry
- Pharmacology
- Earth science
- Geography
- Geology
- Astronomy
- Physics
- Agriculture
- Agricultural Studies
- Computer Science
- Internet
- IT Management
- Web Design
- Mathematics
- Business
- Accounting
- Finance
- Investments
- Logistics
- Trade
- Management
- Marketing
- Engineering and Technology
- Engineering
- Technology
- Aeronautics
- Aviation
- Medicine and Health
- Alternative Medicine
- Healthcare
- Nursing
- Nutrition
- Communications and Media
- Advertising
- Communication Strategies
- Journalism
- Public Relations
- Education
- Educational Theories
- Pedagogy
- Teacher's Career
- Statistics
- Chicago/Turabian
- Nature
- Company Analysis
- Sport
- Paintings
- E-commerce
- Holocaust
- Education Theories
- Fashion
- Shakespeare
- Canadian Studies
- Science
- Food Safety
- Relation of Global Warming and Extreme Weather Condition
Paper Types
- Movie Review
- Essay
- Admission Essay
- Annotated Bibliography
- Application Essay
- Article Critique
- Article Review
- Article Writing
- Assessment
- Book Review
- Business Plan
- Business Proposal
- Capstone Project
- Case Study
- Coursework
- Cover Letter
- Creative Essay
- Dissertation
- Dissertation - Abstract
- Dissertation - Conclusion
- Dissertation - Discussion
- Dissertation - Hypothesis
- Dissertation - Introduction
- Dissertation - Literature
- Dissertation - Methodology
- Dissertation - Results
- GCSE Coursework
- Grant Proposal
- Admission Essay
- Annotated Bibliography
- Application Essay
- Article
- Article Critique
- Article Review
- Article Writing
- Assessment
- Book Review
- Business Plan
- Business Proposal
- Capstone Project
- Case Study
- Coursework
- Cover Letter
- Creative Essay
- Dissertation
- Dissertation - Abstract
- Dissertation - Conclusion
- Dissertation - Discussion
- Dissertation - Hypothesis
- Dissertation - Introduction
- Dissertation - Literature
- Dissertation - Methodology
- Dissertation - Results
- Essay
- GCSE Coursework
- Grant Proposal
- Interview
- Lab Report
- Literature Review
- Marketing Plan
- Math Problem
- Movie Analysis
- Movie Review
- Multiple Choice Quiz
- Online Quiz
- Outline
- Personal Statement
- Poem
- Power Point Presentation
- Power Point Presentation With Speaker Notes
- Questionnaire
- Quiz
- Reaction Paper
- Research Paper
- Research Proposal
- Resume
- Speech
- Statistics problem
- SWOT analysis
- Term Paper
- Thesis Paper
- Accounting
- Advertising
- Aeronautics
- African-American Studies
- Agricultural Studies
- Agriculture
- Alternative Medicine
- American History
- American Literature
- Anatomy
- Anthropology
- Antique Literature
- APA
- Archaeology
- Architecture
- Art
- Asian History
- Asian Literature
- Astronomy
- Aviation
- Biology
- Business
- Canadian Studies
- Chemistry
- Chicago/Turabian
- Classic English Literature
- Communication Strategies
- Communications and Media
- Company Analysis
- Computer Science
- Creative Writing
- Criminal Justice
- Dance
- Design
- Drama
- E-commerce
- Earth science
- East European Studies
- Ecology
- Economics
- Education
- Education Theories
- Educational Theories
- Engineering
- Engineering and Technology
- English
- Ethics
- Family and Consumer Science
- Fashion
- Finance
- Food Safety
- Geography
- Geology
- Harvard
- Healthcare
- High School
- History
- Holocaust
- Internet
- Investments
- IT Management
- Journalism
- Latin-American Studies
- Law
- Legal Issues
- Linguistics
- Literature
- Logistics
- Management
- Marketing
- Master's
- Mathematics
- Medicine and Health
- MLA
- Movies
- Music
- Native-American Studies
- Natural Sciences
- Nature
- Nursing
- Nutrition
- Painting
- Paintings
- Pedagogy
- Pharmacology
- PhD
- Philosophy
- Physics
- Political Science
- Psychology
- Public Relations
- Relation of Global Warming and Extreme Weather Condition
- Religion
- Science
- Shakespeare
- Social Issues
- Social Work
- Sociology
- Sport
- Statistics
- Teacher's Career
- Technology
- Theatre
- Theology
- Tourism
- Trade
- Undergraduate
- Web Design
- West European Studies
- Women and Gender Studies
- World Affairs
- World Literature
- Zoology
Machine Learning Applications in Cancer Prognosis and Prediction, Coursework Example
Hire a Writer for Custom Coursework
Use 10% Off Discount: "custom10" in 1 Click 👇
You are free to use it as an inspiration or a source for your own work.
The data retrieved in the following report breaks down how decision support is scalable in a clinical setting and ultimately results in improved performance as well as patient outcomes. More importantly, it establishes an environment where furthering evidence based practice is more probable in clinical setting. Practitioner performance and patient outcomes significantly improve practitioner performance and that through clinical decisions support systems (CDSSs) a diverse array of decision support modes are offered in which drug prescribing, informed suggestions for active care issues, and existing health issues are critiqued. The following will show how this ultimately results in a clinical environment where decision support improves patient outcomes.
In Peacock et al. (2014), the authors assesses clinical decision making and the way it’s impacted through the enhancement of differentia diagnosis and patient exposure in the basic science classroom. The authors argue that when any real patient is introduced into a pathology classroom there is invaluable knowledge gained that serve as support for clinical decision making. The authors further point out that integrating the type of data that comes from introducing real patients into these classroom environments forms the fundamental principles for medical history, disease pathology with clinical presentation. The methodology of the study utilizes three patients each with their own selective pathology. The work is similar to the studies of Reyna et al. (2015), where the authors make a similar connection between patient pathologies, decisions support and cancer. In their work on decision making and cancer they, “review decision making along the cancer continuum in the contemporary context of informed and shared decision making in which patients are encouraged to take a more active role in their health care” Reyna et al. (2015). Peacock et al.’s study revealed that clinical decision making skills were dramatically enhanced, when real patients were introduced n these settings and data driven pathologies were used. In a similar study by Kourou et al., (2015) assesses machine learning applications in cancer prognosis and prediction. In the field of computational and structural biotechnology, the authors find that Cancer has been characterized as a heterogeneous disease which consists of many different subtypes and through decisions support, a broader scale of data can be provided to each practitioner, which enables him to make a more acute diagnosis and ultimately improves the patient outcome. The early diagnosis and prognosis of a cancer type have become a necessity in cancer research, as it can facilitate the subsequent clinical management of patients.
In Garg et al (2005), assess the effects of computerized clinical decision support systems and the way they impact practitioner performance. The context of their study revolves around software developers for the healthcare industry and their main focus involves improvements based on clinical decision support systems (CDSS). They attribute improvements in patient care and patient outcomes to the use of CDSS. The study reviews controlled trials assessing the impact computerized clinical decision support systems (CDSSs) have on patient outcomes. The authors note that they retrieve their data through the use of EMBASE, MEDLINE, Cochrane Inspec, Library, and ISI databases where they retrieve documented research from 64 reports on the topic. Within the study the authors include non-randomized as well as randomized studies to assess the difference between care provided with CDSS and care provided without it. They primarily focus on patient outcomes and practitioner performance, noting that, “the CDSS improved practitioner performance in 62 (64%) of the 97 studies assessing this outcome, including 4 (40%) of 10 diagnostic systems, 16 (76%) of 21 reminder systems, 23 (62%) of 37 disease management systems, and 19 (66%) of 29 drug-dosing or prescribing systems. Fifty-two trials assessed 1 or more patient outcomes, of which 7 trials (13%) reported improvements” (Garg et al, 2005). The study finds that practitioner performance and patient outcomes significantly improved practitioner performance, and that through CDSSs a variety of decision support modes are offered in which drug prescribing, informed suggestions for active care issues, and existing health issues are critiqued.
In Bates et al. (2003), the authors talk about the Ten Commandments required for effective use of clinical decision support, when attempting to utilize evidence based medicine in real clinical settings. The authors note that despite the fact there is a wide range of support for evidence-based medicine in the healthcare industry, there are some complications in respect to getting physicians to actually practice it. The authors note that, “across most domains in medicine, practice has lagged behind knowledge by at least several years. The authors believe that the key tools for closing this gap will be information systems that provide decision support to users at the time they make decisions, which should result in improved quality of care” (Bates et al., 2003). They further point out that healthcare providers perform a variety of services that are vulnerable to medical errors where clinical decisions aren’t made properly. They identify clinical decision support as an ideal path towards ensuring the work gets done and that errors are reduced. Over the past eight years, the authors have assessed impact of decision support across a broad array of domains and have found a number of common elements important to success. The goal of this report is to discuss these lessons learned in the interest of informing the efforts of others working to make the practice of evidence-based medicine a reality. The authors identify ten key commandments of clinical decision support, noting that “1) Speed is everything, 2) predict preemptive needs and satisfy them in real time, 3) Fit into the User’s Workflow, 4) Little Things Can Make a Big Difference” (Bates et al., 2003). The authors further note for commandment 5 that it must be recognized that physicians are going to resist intervention in the decision support process. They further point out that in the prognosis and treatment process, changing direction can be a favored alternative to stopping and the study identifies this as commandment 6. The authors further point out that commandments seven through ten entail “7) Simple Interventions Work Best, 8) that clinicians should ask for additional information, but only when it’s needed, 9)”Monitor impact, Get Feedback, and Respond, 10) Manage and Maintain your knowledge-based systems” (Bates et al., 2003). The primary finding of the study is that through decision support and following these commandments, significant improvements in patient outcomes and physician performance can be achieved, but most importantly this can present a better approach to evidence based practice.
Kawamoto et al. (2005) provide information on how clinical practices improve through the use of decision support. The authors orchestrate a systematic review of randomized controlled trials assessing the 15 decision support system features. Their results uncover the fact that in 68% of the trials where decision support systems were used clinical practice and patient outcomes were improved. The authors note that, “univariate analyses revealed that, for five of the system features, interventions possessing the feature were significantly more likely to improve clinical practice than interventions lacking the feature. Multiple logistic regression analysis identified four features as independent predictors of improved clinical practice” (Kawamoto et al., 2005). The study further pointed out that of the 32 systems evaluated, 30 (94%) reveal that there was a substantial improvement in patient outcomes and clinical practices. It was further found that there was a direct experimental incentive for providing periodic performance feedback, which shared recommendations with patients, and requesting documentation of reasons for not following recommendations.
Tsoukalas et al. (2015), assesses the value of data to optimal decision making, finding that data-driven, probabilistic machine learning in clinical settings is an ideal method of decision support, specifically for patients with sepsis. The ability to extract informed decisions from this method is identified as being much more probable than traditional methods of evidence based practice. As the authors note, “we present a data-driven, probabilistic framework for clinical decision support in sepsis-related cases. We first define states, actions, observations and rewards based on clinical practice, expert knowledge and data representations in an EHR dataset of 1492 patients” (Tsoukalas et al., 2015). Through the use of this wide data set, the authors have a substantial number of patients, from which they can draw their research. The primary objective of the study focused on policy, specifically as it related to implementation and use of policy where discussion support served as an integral part of the practitioner’s work environment. In their study the authors note that, “when the optimal policy was followed, 387 patients (25.9%) have 90% of their transitions to better states and 503 patients (33.7%) patients had 90% of their transitions to worse states (P=4.0e-06), while in the non-policy cases, these numbers are 192 (12.9%) and 764 (51.2%) patients (P=4.6e-117), respectively” (Tsoukalas et al., 2015). The data-driven model provided by these authors basically reveals that decision support is a practice that enhances the ability of clinical practitioners to predict mortality to a much more distinct accuracy. Their research reveals that through the use of decisions support, clinical practitioners can better treat patients as well as further knowledge in the field regarding treatment.
Conclusion
In sum the data retrieved in this report reveals that decision support is scalable in a clinical setting and ultimately results in improved performance as well as patient outcomes. Most importantly it creates an environment where furthering evidence based practice is more probable in clinical setting. Practitioner performance and patient outcomes significantly improved practitioner performance, and that through CDSSs a variety of decision support modes are offered in which drug prescribing, informed suggestions for active care issues, and existing health issues are critiqued. This ultimately results in a clinical environment where decision support improves patient outcomes.
References
Bates, D. W., Kuperman, G. J., Wang, S., Gandhi, T., Kittler, A., Volk, L., … & Middleton, B. (2003). Ten commandments for effective clinical decision support: making the practice of evidence-based medicine a reality. Journal of the American Medical Informatics Association, 10(6), 523-530.
Garg, A. X., Adhikari, N. K., McDonald, H., Rosas-Arellano, M. P., Devereaux, P. J., Beyene, J., … & Haynes, R. B. (2005). Effects of computerized clinical decision support systems on practitioner performance and patient outcomes: a systematic review. Jama, 293(10), 1223-1238.
Kawamoto, K., Houlihan, C. A., Balas, E. A., & Lobach, D. F. (2005). Improving clinical practice using clinical decision support systems: a systematic review of trials to identify features critical to success. Bmj, 330(7494), 765.
Kourou, K., Exarchos, T. P., Exarchos, K. P., Karamouzis, M. V., & Fotiadis, D. I. (2015). Machine learning applications in cancer prognosis and prediction. Computational and Structural Biotechnology Journal, 13, 8-17.
Peacock, J. G., & Grande, J. P. (2014). Patient exposure in the basic science classroom enhances differential diagnosis formation and clinical decision-making (No. e744v1). PeerJ PrePrints.
Reyna, V. F., Nelson, W. L., Han, P. K., & Pignone, M. P. (2015). Decision making and cancer. American Psychologist, 70(2), 105.
Tsoukalas, A., Albertson, T., & Tagkopoulos, I. (2015). From Data to Optimal Decision Making: A Data-Driven, Probabilistic Machine Learning Approach to Decision Support for Patients With Sepsis. JMIR Medical Informatics, 3(1), e11.
Stuck with your Coursework?
Get in touch with one of our experts for instant help!
Tags:
Time is precious
don’t waste it!
writing help!
Plagiarism-free
guarantee
Privacy
guarantee
Secure
checkout
Money back
guarantee
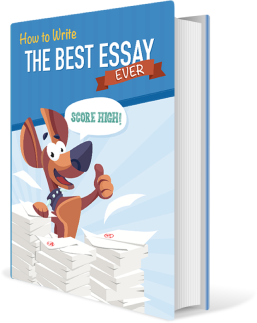