Disciplines
- MLA
- APA
- Master's
- Undergraduate
- High School
- PhD
- Harvard
- Biology
- Art
- Drama
- Movies
- Theatre
- Painting
- Music
- Architecture
- Dance
- Design
- History
- American History
- Asian History
- Literature
- Antique Literature
- American Literature
- Asian Literature
- Classic English Literature
- World Literature
- Creative Writing
- English
- Linguistics
- Law
- Criminal Justice
- Legal Issues
- Ethics
- Philosophy
- Religion
- Theology
- Anthropology
- Archaeology
- Economics
- Tourism
- Political Science
- World Affairs
- Psychology
- Sociology
- African-American Studies
- East European Studies
- Latin-American Studies
- Native-American Studies
- West European Studies
- Family and Consumer Science
- Social Issues
- Women and Gender Studies
- Social Work
- Natural Sciences
- Anatomy
- Zoology
- Ecology
- Chemistry
- Pharmacology
- Earth science
- Geography
- Geology
- Astronomy
- Physics
- Agriculture
- Agricultural Studies
- Computer Science
- Internet
- IT Management
- Web Design
- Mathematics
- Business
- Accounting
- Finance
- Investments
- Logistics
- Trade
- Management
- Marketing
- Engineering and Technology
- Engineering
- Technology
- Aeronautics
- Aviation
- Medicine and Health
- Alternative Medicine
- Healthcare
- Nursing
- Nutrition
- Communications and Media
- Advertising
- Communication Strategies
- Journalism
- Public Relations
- Education
- Educational Theories
- Pedagogy
- Teacher's Career
- Statistics
- Chicago/Turabian
- Nature
- Company Analysis
- Sport
- Paintings
- E-commerce
- Holocaust
- Education Theories
- Fashion
- Shakespeare
- Canadian Studies
- Science
- Food Safety
- Relation of Global Warming and Extreme Weather Condition
Paper Types
- Movie Review
- Essay
- Admission Essay
- Annotated Bibliography
- Application Essay
- Article Critique
- Article Review
- Article Writing
- Assessment
- Book Review
- Business Plan
- Business Proposal
- Capstone Project
- Case Study
- Coursework
- Cover Letter
- Creative Essay
- Dissertation
- Dissertation - Abstract
- Dissertation - Conclusion
- Dissertation - Discussion
- Dissertation - Hypothesis
- Dissertation - Introduction
- Dissertation - Literature
- Dissertation - Methodology
- Dissertation - Results
- GCSE Coursework
- Grant Proposal
- Admission Essay
- Annotated Bibliography
- Application Essay
- Article
- Article Critique
- Article Review
- Article Writing
- Assessment
- Book Review
- Business Plan
- Business Proposal
- Capstone Project
- Case Study
- Coursework
- Cover Letter
- Creative Essay
- Dissertation
- Dissertation - Abstract
- Dissertation - Conclusion
- Dissertation - Discussion
- Dissertation - Hypothesis
- Dissertation - Introduction
- Dissertation - Literature
- Dissertation - Methodology
- Dissertation - Results
- Essay
- GCSE Coursework
- Grant Proposal
- Interview
- Lab Report
- Literature Review
- Marketing Plan
- Math Problem
- Movie Analysis
- Movie Review
- Multiple Choice Quiz
- Online Quiz
- Outline
- Personal Statement
- Poem
- Power Point Presentation
- Power Point Presentation With Speaker Notes
- Questionnaire
- Quiz
- Reaction Paper
- Research Paper
- Research Proposal
- Resume
- Speech
- Statistics problem
- SWOT analysis
- Term Paper
- Thesis Paper
- Accounting
- Advertising
- Aeronautics
- African-American Studies
- Agricultural Studies
- Agriculture
- Alternative Medicine
- American History
- American Literature
- Anatomy
- Anthropology
- Antique Literature
- APA
- Archaeology
- Architecture
- Art
- Asian History
- Asian Literature
- Astronomy
- Aviation
- Biology
- Business
- Canadian Studies
- Chemistry
- Chicago/Turabian
- Classic English Literature
- Communication Strategies
- Communications and Media
- Company Analysis
- Computer Science
- Creative Writing
- Criminal Justice
- Dance
- Design
- Drama
- E-commerce
- Earth science
- East European Studies
- Ecology
- Economics
- Education
- Education Theories
- Educational Theories
- Engineering
- Engineering and Technology
- English
- Ethics
- Family and Consumer Science
- Fashion
- Finance
- Food Safety
- Geography
- Geology
- Harvard
- Healthcare
- High School
- History
- Holocaust
- Internet
- Investments
- IT Management
- Journalism
- Latin-American Studies
- Law
- Legal Issues
- Linguistics
- Literature
- Logistics
- Management
- Marketing
- Master's
- Mathematics
- Medicine and Health
- MLA
- Movies
- Music
- Native-American Studies
- Natural Sciences
- Nature
- Nursing
- Nutrition
- Painting
- Paintings
- Pedagogy
- Pharmacology
- PhD
- Philosophy
- Physics
- Political Science
- Psychology
- Public Relations
- Relation of Global Warming and Extreme Weather Condition
- Religion
- Science
- Shakespeare
- Social Issues
- Social Work
- Sociology
- Sport
- Statistics
- Teacher's Career
- Technology
- Theatre
- Theology
- Tourism
- Trade
- Undergraduate
- Web Design
- West European Studies
- Women and Gender Studies
- World Affairs
- World Literature
- Zoology
An Exercise in Logistic Regression, Essay Example
Hire a Writer for Custom Essay
Use 10% Off Discount: "custom10" in 1 Click 👇
You are free to use it as an inspiration or a source for your own work.
Abstract
This paper examined building a predictive model for understanding which consumers may potentially default on bank-sponsored loans. The paper builds three different models based on the variables given in “bank loan.xls”; a more parsimonious model is selected in order to protect against multicollinearity and bias in the model. Once the model is selected, it is applied to a group of potential loan consumers that are considered to be “high risk” for the bank. Finally, three different academic papers are examined to understand how different logistic regression models may be used in different academic disciplines.
Introduction
This paper deals with logistic regression in two different ways. First, a statistical model is built based on historical data from a bank regarding loan consumers. The logistic regression model identifies key variables that may be useful in predicting which consumers are default risks. Once the model is finished, it is applied to a data file of 150 potential loan consumers. Finally, three different academic papers are examined to see how different logistic regression models may be built.
Data Analysis
The data set provided for analysis was “bank loans. xls.” The data set is separated into two different segments: 1) a list of 700 potential consumers seeking bank loans; 2) a list of 150 consumers that already received bank loans. The point of the exercise is to first, analyze a sample of the 700 potential consumers in order to create a predictive model of loan default. Once that model is established, it will be “back tested” against the historical record of 150 consumers to determine its ultimate accuracy.
To begin the analysis, a sample of 300 potential consumers was selected from the original database of 700 consumers (numbered 1-300). Before analyzing the model, however, the correlations of the variables were looked at in order to identify the presence of multicollinearity. Multicollinearity occurs when two or more variables capture the same data, and thus tend to result in high error levels and inaccurate variable coefficients. In Appendix one, the correlation values for the variables is listed. While employment is potentially a proxy used for income, both variables will be left in the model because employment expresses the length of a working career (not merely indicating employment status) and income is paramount in understanding one’s ability to repay a loan. There were also questions about whether all three measures of debt and three measures of predef are necessary in the model or if only a proxy for those variables was necessary.
In order to sort out whether multicollinearity might be a problem or not, two different models were run. “Model A” ran all variables in the model; “Model B” removed predef (1-3) but kept in three variables for debt; “Model C” chose total debt as a proxy for debt. Looking at the results in Appendix 1, the main cause for concern in Model A and Model B was that variables income and debt, normally viewed as independent predictors of credit, are not significant. In Model C, once the proxies are accounted for, income and debtinc are highly significant predictors. Thus, Model C was selected as the final model to analyze with the final variables: Age, education level (categorical variable with four different indicator variables), employment, address, income, debtinc. Although the model was significant, the independent predictors were income, debtinc, and indicator variables related to education. The dependent variable in the analysis was “default”, a dichotomous variable.
The variables were initially put into the model all at once retaining them over the course of analysis (enter method). Looking at Appendix 1, the model selected was able to predict correctly in 76.6% of cases. The ability of the model to explain variance in defaults, however, was not impressive: the two “r-squared” statistics show that the model explains from 20% to 30% of variance in the model.
Using the model built above, the 150 potential loan consumers were tested to see if they were good risks. Based on the averages of the individuals involved in the areas covered in model c (age, education level, employment, address, income, debtinc), the individuals were not considered to be good risks as their average stats are similar to those who defaulted in the larger data set.
Literature Review
There are a total of three academic papers that use multivariate logistic regression. Simnett et al. explore the question of why firms choose to assure (essentially an audit) sustainability report. In particular, the authors identity two sets of hypotheses to test the question: Set 1) Companies with a greater need to increase confidence will be more likely to have their reports assured and assured from the auditing profession; Set 2) Companies domiciled in countries that are more stakeholder-oriented are more likely to demand assurance with companies in a less shareholder-oriented environment and choose it from the auditing profession.
In order to model this relationship, Simnett chose logistic regression in order to test the relationships.
Afroza et al. explore the relationship between firm size and the propensity for merger and acquisition activity in the European financial sector. In particular, four hypotheses were tested in this study: 1) Firm size is positively related to the probability that the firm will become an acquirer; 2) Firm size is negatively related to the probability that the firm will be acquired or participate in a merger; 3) Well-managed institutions are more likely to be acquirers; 4) Poorly managed institutions are more likely to be acquired (Simnett et al, 55). In order to test the model, the authors tested a model looking at the likelihood that a European institution had participated in mergers or acquisitions during the period 1995-2001 with the variables: Assets; return on equity, efr costs, loans, non-financing, deposits, capital, domcred (Simnett et al, 56).
Unlike most dependent variables in logit analysis that are dichotomous in nature, the dependent variable in this analysis is divided into four different responses: “0” for no involvement in 1995-2001; “1” if it was announced in the following year (n+1) that the institution acquired another; “2” if it was announced that the institution was acquired by another European credit institution; “3” if it was announced that the institution participated in a merger (Simnett et al., 57).
Overall, the results illustrated that the size of the firm was a predictor of the acquiring institution based on the positive, significant coefficient of the variable “assets.” “ASSET” was also significant in proving the second hypothesis. In order to assess the second hypothesis, the quality of management was measured using return on equity and cost efficiency ratio. Due to the low level of statistical significance (above 10%), the hypothesis was not proven. Overall, the paper illustrated that size is a key variable in establishing whether a firm will acquire another.
Ucbasaran et al. explore the role of human capital in the development of entrepreneurs. The authors, in order test a total of six hypotheses, break down the concept of “human” capital into different components. Indeed, in order to measure an entrepreneur’s human capital, education and work experience are identified as the main proxies for “general” human capital; prior business experience and self- perceived capabilities are considered as proxies of “entrepreneurship” human capital (Ucbasaran et al., 155).
From this initial conceptualization of human capital, the authors come up with six different hypotheses to identify which are the most important in the development of entrepreneurs. The dependent variables in the model were based on the number of opportunities the entrepreneur had to start a business; the dependent variable, like other models above, was transformed into a categorical variable: “1” for entrepreneurs who were unable to identify opportunities; “2” for entrepreneurs that identified one or two opportunities; “3” for entrepreneurs that had identified more than three opportunities (Ucbasaran et al., 160). There were a number of independent variables chosen to operationalize the concepts of education, work experience, business work experience, etc. To test the hypotheses, the authors built five different logit models: one model composed of control variables; one model composed of general human capital and control variables; one model of entrepreneurship specific human capital and control variables; and one model that combine all models into one.
Overall, while the relationship between human capital and opportunities has not been explored, the study showed that entrepreneur specific human capital skills were important in obtaining the number of opportunities.
References:
Azofra, S.S., Myriam, G.O., Begona, T. (2008). Size, Targer Performance and European Bank Mergers and Acquisition. American Journal of Business, 23(1), 53-63.
Simnett, R., Vanstraelen, A. & Chua, W.F. (2009). Assurance on sustainability reports: an international comparison. The Accounting Review. 84(3), 937-967.
Ucbasaran, D., Westhead, P. & Wright, M. (2008). Opportunity Identification and Pursuit: Does an Entrepeneur’s Human Capital Matter? Small Business Economics, 30(2), 153-173.
Appendix 1
Correlations |
|||||||||||||||
age | educationlevel | employment | address | income | |||||||||||
age | Pearson Correlation | 1 | .034 | .539** | -.197** | .517** | |||||||||
Sig. (2-tailed) | .554 | .000 | .001 | .000 | |||||||||||
N | 299 | 299 | 299 | 299 | 299 | ||||||||||
educationlevel | Pearson Correlation | .034 | 1 | -.176** | .102 | .202** | |||||||||
Sig. (2-tailed) | .554 | .002 | .077 | .000 | |||||||||||
N | 299 | 299 | 299 | 299 | 299 | ||||||||||
employment | Pearson Correlation | .539** | -.176** | 1 | -.073 | .676** | |||||||||
Sig. (2-tailed) | .000 | .002 | .208 | .000 | |||||||||||
N | 299 | 299 | 299 | 299 | 299 | ||||||||||
address | Pearson Correlation | -.197** | .102 | -.073 | 1 | -.049 | |||||||||
Sig. (2-tailed) | .001 | .077 | .208 | .403 | |||||||||||
N | 299 | 299 | 299 | 299 | 299 | ||||||||||
income | Pearson Correlation | .517** | .202** | .676** | -.049 | 1 | |||||||||
Sig. (2-tailed) | .000 | .000 | .000 | .403 | |||||||||||
N | 299 | 299 | 299 | 299 | 299 | ||||||||||
debtinc | Pearson Correlation | .001 | .058 | -.065 | .036 | -.078 | |||||||||
Sig. (2-tailed) | .993 | .317 | .266 | .531 | .177 | ||||||||||
N | 299 | 299 | 299 | 299 | 299 | ||||||||||
creddebt | Pearson Correlation | .278** | .119* | .395** | .029 | .555** | |||||||||
Sig. (2-tailed) | .000 | .041 | .000 | .614 | .000 | ||||||||||
N | 299 | 299 | 299 | 299 | 299 | ||||||||||
othdebt | Pearson Correlation | .322** | .131* | .388** | -.013 | .525** | |||||||||
Sig. (2-tailed) | .000 | .024 | .000 | .824 | .000 | ||||||||||
N | 299 | 299 | 299 | 299 | 299 | ||||||||||
VAR00013 | Pearson Correlation | -.385** | .212** | -.592** | .065 | -.282** | |||||||||
Sig. (2-tailed) | .000 | .000 | .000 | .264 | .000 | ||||||||||
N | 299 | 299 | 299 | 299 | 299 | ||||||||||
VAR00014 | Pearson Correlation | -.286** | .241** | -.573** | .032 | -.262** | |||||||||
Sig. (2-tailed) | .000 | .000 | .000 | .577 | .000 | ||||||||||
N | 299 | 299 | 299 | 299 | 299 | ||||||||||
VAR00015 | Pearson Correlation | -.001 | .064 | -.050 | .037 | -.073 | |||||||||
Sig. (2-tailed) | .987 | .270 | .387 | .526 | .208 | ||||||||||
N | 299 | 299 | 299 | 299 | 299 | ||||||||||
B | S.E. | Wald | df | Sig. | Exp(B) | ||||||||||
Step 1a | age | .008 | .024 | .107 | 1 | .744 | 1.008 | ||||||||
educationlevel | 6.456 | 3 | .091 | ||||||||||||
educationlevel(1) | 1.766 | .902 | 3.830 | 1 | .050 | 5.848 | |||||||||
educationlevel(2) | 1.970 | .888 | 4.919 | 1 | .027 | 7.172 | |||||||||
educationlevel(3) | 1.070 | .970 | 1.216 | 1 | .270 | 2.915 | |||||||||
employment | -.231 | .050 | 21.559 | 1 | .000 | .794 | |||||||||
address | -.084 | .061 | 1.925 | 1 | .165 | .919 | |||||||||
income | .012 | .016 | .589 | 1 | .443 | 1.012 | |||||||||
debtinc | .088 | .051 | 2.992 | 1 | .084 | 1.092 | |||||||||
creddebt | .355 | .170 | 4.366 | 1 | .037 | 1.426 | |||||||||
othdebt | -.035 | .136 | .065 | 1 | .799 | .966 | |||||||||
Constant | -3.111 | 1.322 | 5.535 | 1 | .019 | .045 | |||||||||
Classification Tablea,b | |||||
Observed | Predicted | ||||
default | Percentage Correct | ||||
0 | 1 | ||||
Step 0 | default | 0 | 229 | 0 | 100.0 |
1 | 70 | 0 | .0 | ||
Overall Percentage | 76.6 | ||||
a. Constant is included in the model.
b. The cut value is .500
|
Model Summary | |||
Step | -2 Log likelihood | Cox & Snell R Square | Nagelkerke R Square |
1 | 258.697a | .200 | .302 |
a. Estimation terminated at iteration number 5 because parameter estimates changed by less than .001.
|
Model A
Model B
Variables in the Equation |
|||||||||||||||||||||||||||||||||||||||||||||||||||||||||||||||||||||||||||||||||||||||||||||||||||||||||||||||||||||||||||
B | S.E. | Wald | df | Sig. | Exp(B) | ||||||||||||||||||||||||||||||||||||||||||||||||||||||||||||||||||||||||||||||||||||||||||||||||||||||||||||||||||||||
Step 1a | age | .008 | .024 | .107 | 1 | .744 | 1.008 | ||||||||||||||||||||||||||||||||||||||||||||||||||||||||||||||||||||||||||||||||||||||||||||||||||||||||||||||||||||
educationlevel | 6.456 | 3 | .091 | ||||||||||||||||||||||||||||||||||||||||||||||||||||||||||||||||||||||||||||||||||||||||||||||||||||||||||||||||||||||||
educationlevel(1) | 1.766 | .902 | 3.830 | 1 | .050 | 5.848 | |||||||||||||||||||||||||||||||||||||||||||||||||||||||||||||||||||||||||||||||||||||||||||||||||||||||||||||||||||||
educationlevel(2) | 1.970 | .888 | 4.919 | 1 | .027 | 7.172 | |||||||||||||||||||||||||||||||||||||||||||||||||||||||||||||||||||||||||||||||||||||||||||||||||||||||||||||||||||||
educationlevel(3) | 1.070 | .970 | 1.216 | 1 | .270 | 2.915 | |||||||||||||||||||||||||||||||||||||||||||||||||||||||||||||||||||||||||||||||||||||||||||||||||||||||||||||||||||||
employment | -.231 | .050 | 21.559 | 1 | .000 | .794 | |||||||||||||||||||||||||||||||||||||||||||||||||||||||||||||||||||||||||||||||||||||||||||||||||||||||||||||||||||||
address | -.084 | .061 | 1.925 | 1 | .165 | .919 | |||||||||||||||||||||||||||||||||||||||||||||||||||||||||||||||||||||||||||||||||||||||||||||||||||||||||||||||||||||
income | .012 | .016 | .589 | 1 | .443 | 1.012 | |||||||||||||||||||||||||||||||||||||||||||||||||||||||||||||||||||||||||||||||||||||||||||||||||||||||||||||||||||||
debtinc | .088 | .051 | 2.992 | 1 | .084 | 1.092 | |||||||||||||||||||||||||||||||||||||||||||||||||||||||||||||||||||||||||||||||||||||||||||||||||||||||||||||||||||||
creddebt | .355 | .170 | 4.366 | 1 | .037 | 1.426 | |||||||||||||||||||||||||||||||||||||||||||||||||||||||||||||||||||||||||||||||||||||||||||||||||||||||||||||||||||||
othdebt | -.035 | .136 | .065 | 1 | .799 | .966 | |||||||||||||||||||||||||||||||||||||||||||||||||||||||||||||||||||||||||||||||||||||||||||||||||||||||||||||||||||||
Constant | -3.111 | 1.322 | 5.535 | 1 | .019 | .045 | |||||||||||||||||||||||||||||||||||||||||||||||||||||||||||||||||||||||||||||||||||||||||||||||||||||||||||||||||||||
a. Variable(s) entered on step 1: age, educationlevel, employment, address, income, debtinc, creddebt, othdebt.
|
|||||||||||||||||||||||||||||||||||||||||||||||||||||||||||||||||||||||||||||||||||||||||||||||||||||||||||||||||||||||||||
Model C |
Variables in the Equation | |||||||
B | S.E. | Wald | df | Sig. | Exp(B) | ||
Step 1a | age | .001 | .024 | .000 | 1 | .982 | 1.001 |
educationlevel | 7.065 | 3 | .070 | ||||
educationlevel(1) | 1.937 | .926 | 4.379 | 1 | .036 | 6.940 | |
educationlevel(2) | 2.114 | .910 | 5.402 | 1 | .020 | 8.285 | |
educationlevel(3) | 1.210 | .984 | 1.512 | 1 | .219 | 3.354 | |
employment | -.223 | .048 | 21.433 | 1 | .000 | .800 | |
address | -.077 | .060 | 1.626 | 1 | .202 | .926 | |
income | .025 | .010 | 6.485 | 1 | .011 | 1.025 | |
debtinc | .122 | .025 | 23.626 | 1 | .000 | 1.130 | |
Constant | -3.562 | 1.238 | 8.277 | 1 | .004 | .028 | |
a. Variable(s) entered on step 1: age, educationlevel, employment, address, income, debtinc.
|
Stuck with your Essay?
Get in touch with one of our experts for instant help!
Time is precious
don’t waste it!
writing help!
Plagiarism-free
guarantee
Privacy
guarantee
Secure
checkout
Money back
guarantee
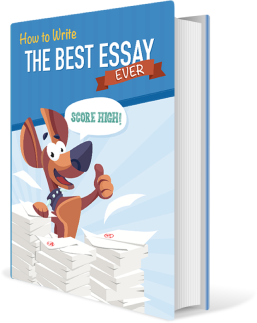